Transforming AI Efficiency with Advanced Data Annotation Tools and Platforms
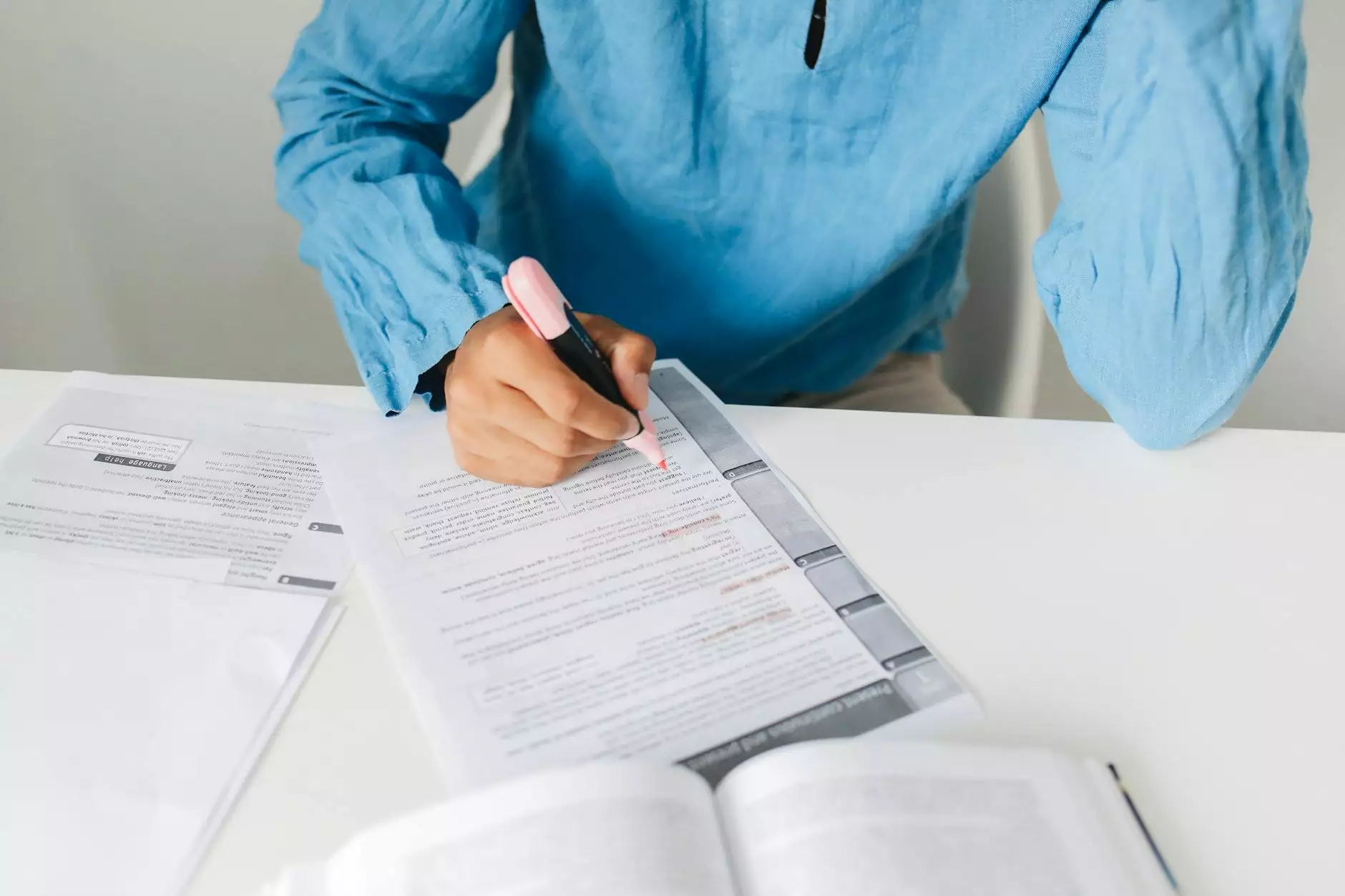
The data annotation tool sector has gained significant importance in recent years as businesses and organizations increasingly rely on artificial intelligence (AI) and machine learning (ML) technologies. These technologies transform raw data into meaningful insights and applications by creating high-quality, labeled datasets that can train algorithms to perform tasks accurately. In this article, we delve into the essentials of data annotation, exploring tools and platforms available for modern businesses. Our aim is to illuminate the critical role of data annotation in AI development and how https://keylabs.ai/ stands at the forefront of this innovation.
Understanding Data Annotation
Data annotation refers to the process of labeling or tagging data, which can include images, videos, text, and audio. By providing context and additional information about the data, annotations enable machine learning models to learn and make predictions based on input data. The effectiveness of data annotations directly influences the performance of AI applications.
Types of Data Annotation
- Image Annotation: Involves labeling objects within images, often used in computer vision applications like facial recognition and autonomous vehicles.
- Text Annotation: Involves tagging words, phrases, or sentences in texts, essential for natural language processing (NLP) tasks like sentiment analysis and chatbots.
- Audio Annotation: Refers to labeling audio files, critical for speech recognition systems and voice-activated assistants.
- Video Annotation: Entails labeling video content frame-by-frame, used in security systems and behavioral analysis.
The Importance of Data Annotation Tools
As AI continues to evolve, the necessity for effective data annotation tools becomes paramount. Businesses rely on these tools to create datasets that are not only vast but also rich in detail and quality. The following are key reasons businesses should invest in robust data annotation tools:
1. Enhanced Accuracy
High-quality annotated data leads to superior model performance. Data annotation tools reduce human error and provide consistent labeling, which is essential for training precise models.
2. Increased Efficiency
Automation features in modern data annotation tools significantly accelerate the labeling process. This efficiency allows teams to focus on critical tasks while the tool handles repetitive work.
3. Scalability
With the growing volume of data, businesses require scalable solutions. Quality data annotation platforms can handle vast datasets, ensuring consistency and speed without compromising quality.
4. Cost-Effectiveness
Investing in data annotation tools can be cost-effective in the long run. Automated annotation reduces the need for extensive manual labor, leading to lower operational costs.
Overview of Key Data Annotation Platforms
There are numerous data annotation platforms available in the market today, each offering distinct features tailored to meet various business needs. Here are a few notable platforms:
1. Keylabs.ai
Keylabs.ai excels in providing a comprehensive data annotation platform that is designed to streamline the AI training process across various sectors. With a user-friendly interface and powerful annotation capabilities, it empowers businesses to convert raw data into AI-ready datasets efficiently.
2. Amazon SageMaker Ground Truth
This platform by Amazon Web Services (AWS) integrates machine learning into the data labeling process, allowing businesses to automate some labeling tasks intelligently. Its robust features help reduce costs and improve annotation accuracy.
3. Labelbox
Labelbox offers a highly intuitive interface and collaborative features that cater to both technical and non-technical users. It supports a wide variety of data types and is recognized for its efficiency in managing the annotation workflow.
4. Supervisely
This platform is particularly geared towards computer vision projects, providing tools for video and image annotations. Supervisely also offers data versioning and visualization features that enhance project management.
Key Features of Effective Data Annotation Tools
The right data annotation tool should possess several essential features that contribute to its effectiveness. Here are some qualities to consider when selecting a data annotation solution:
1. Versatility
The platform should support multiple types of data (text, image, video, and audio) to cater to different business requirements.
2. User-Friendly Interface
A straightforward interface enables users of all skill levels to navigate the annotation process easily, ensuring a smoother workflow.
3. Collaboration Features
Annotation projects often require teamwork. Tools with collaborative features allow multiple users to work on a project, improving overall efficiency.
4. Quality Control Mechanisms
In-built quality assurance features help ensure the accuracy of annotations, providing businesses confidence in their labeled datasets.
5. Integration Capabilities
The ability to integrate with existing workflows and systems is crucial. Effective tools should easily connect with data management platforms and machine learning frameworks.
Best Practices for Data Annotation
1. Define Clear Annotation Guidelines
Establishing comprehensive and clear guidelines helps annotators understand the expectations and ensures consistency across the dataset.
2. Use Pre-trained Models When Possible
Utilizing pre-trained machine learning models can assist in annotating data faster. These models can provide initial labels that human annotators can refine.
3. Implement a Review Process
A robust review process involving multiple levels of scrutiny is vital for maintaining high annotation quality. Regular audits can help identify and rectify inconsistencies.
4. Provide Annotators with Necessary Training
Offering training programs ensures that annotators are well-equipped to handle specific tasks, ultimately improving the quality of their work.
5. Leverage Feedback Loops
Creating feedback mechanisms allows continuous improvement in the annotation process. Incorporating feedback from model performance can help refine future annotation tasks.
The Future of Data Annotation in AI
The future of AI and machine learning is undeniably intertwined with data annotation. As technology progresses, the necessity for high-quality annotated data will continue to grow. We anticipate several trends in the data annotation landscape:
- Increased Automation: The integration of AI into data annotation processes will reduce the manual effort required, leading to faster and more accurate annotations.
- Enhanced Collaboration: Future platforms will likely provide improved collaborative functionalities, allowing teams to work seamlessly across different geographies.
- Focus on Quality Over Quantity: As competitive advantages shift, businesses will prioritize high-quality annotations that provide better training data.
- Integration of Advanced Technologies: Innovations such as AI-assisted labeling, which helps human annotators by suggesting labels, will become more common.
Conclusion
With the rapid advancement of AI technologies, the significance of robust data annotation tools and platforms cannot be overstated. By investing in reliable tools like https://keylabs.ai/, businesses can ensure that they not only keep pace with technological developments but also position themselves for sustained success in the AI-driven future. Quality data annotation is the cornerstone of effective AI training, and it is crucial that organizations recognize its value in achieving their strategic objectives. Embrace the power of advanced data annotation, and unlock new possibilities for your AI initiatives today!